155 - Brian Spiesman - Bee Machine (in English)
Transcript
Andony Melathopoulos: [00:00:00] From the Oregon State University Extension Service this is PolliNation, a podcast that tells the stories of researchers, land managers, and concerned citizens making bold strides to improve the health of pollinators. I'm your host, Dr. Andony Melathopoulos, Assistant Professor in Pollinator Health in the Department of Horticulture. There are many things that delight me in this world, but one thing in particular has come to my attention is that there's a lot of interest among regular people in identifying insects and knowing more about their lives. And this has really exploded when it comes to pollinating insects.
[00:00:47] Now we know from previous episodes is particularly tricky identifying some of our pollinators, some like butterflies are little less difficult, but the bees are notoriously difficult to determine to species. [00:01:00] As we heard on a previous episode with Dr. John Ascher, there's a lot of new technology that is coming online, making it easier for people without formal training to be able to get into this gig. And today I'm really delighted to feature one of the innovations that's coming online, with Dr. Brian Spiesmann.
[00:01:19] Now he's in the process of developing a machine learning mechanism that will identify bees, starting with bumblebees. And in this episode, you're going to hear that he's looking for you to use this tool, which you can find a linked in our show notes. And by getting lots of submissions in, he is going to train his algorithms to be able to do really remarkable work identifying bees - helping people who don't have the skills to really participate in bee surveying.
[00:01:46] I also just want to make a shout out we are still doing our podcast survey, this is a survey that helps us improve the podcastcast. You can find it at our website, [00:02:00] pollinationpodcasts.oregonstate.edu. For people who submit survey results, we do send out a pack, which has got a ball cap, and also a book for identification. And I want to announce that Corey Docho, I hope it pronounced your name right in Carnation, Washington is our current winner. So Corey it's on its way out to you, thanks for taking time to complete the survey. And we're going to conclude the survey at the end of October. So please get your survey results in, it really helps us out.
[00:02:28] And now, without further ado, let's talk about Bee Machine with Dr. Brian Spiesmann today on PolliNation!
[00:02:45] So on a previous episode we had with John Ascher, he made the remark that bee research is on the cusp of where birding was in the 1970's. We're sort of starting to move into the realm of these highly motivated [00:03:00] non-professionals who are kind of collecting bee records. Do you agree with this assessment? And tell us a little bit about your personal experiences working with enthusiastic native bee volunteers.
[00:03:12] Brian Spiesmann: [00:03:12] Yeah. So I do agree with it! I think it's a good observation. I think recently there's been a lot of interest in bees and pollinators in general, and to me it seems like it's coming a lot from you know, some of the troubles we've been seeing with honeybees. And I think this acts as sort of a gateway to the broader field of pollinators and bees in general. And so a lot of people once they start realizing that bees are more than just honeybees that there's 4,000 species of native bees in North America - people start to really get excited about that.
[00:03:57] "Wow, I didn't know there was that many bees!" And [00:04:00] now that there are these online resources where people can post their pictures, ask questions things like iNaturalist, there’s Facebook groups where people can get involved with there's Bumblebee Watch. There's some other sort of citizen science or community science organizations where people can get really involved and contribute and they can feel like they're contributing to some of these really important data sets for monitoring bees and other organisms across the United States and in North America. So yeah, I think it's sort of starting to see that broad interest and you can see it in sort of popular culture. So I think that it's going to be really good for creating interest and driving policy [00:05:00] changes that are needed to combat a lot of the pollinator decline and bee decline in particular.
[00:05:06] Andony Melathopoulos: [00:05:06] Yeah, it's true. You know, I noticed that there's a number of Facebook groups that are devoted to just people posting pictures of native bees. And it's really wonderful because people from right across the US are turning up some remarkable bees that they're running into. And they're seeing there's a lot of eyes that are really looking and are getting increasingly sophisticated to looking for bees.
[00:05:30] Brian Spiesmann: [00:05:30] Yeah, definitely. And these observations are really important, especially for tracking endangered species like the rusty patch bumblebee and trying to understand where the range is currently. Is it contracting still, is it expanding? Are we seeing new pockets and new populations in places where we haven't seen them before? Or places where we haven't seen them in a long time?
[00:05:57] Also just for [00:06:00] monitoring those common species, it's important to still continue to see where Bombus impatiens the common eastern bumblebee, one of the most, if not the most common species in North America. If we start to see declines in observations, then that's a real important signal that we need to see. It's the whole gambit of the high diversity of bees all over the world that we need to be able to monitor more effectively. And we really wouldn't be able to do it with a lot of these enthusiasts that are contributing observations from literally all over the world.
[00:06:43] Andony Melathopoulos: [00:06:43] Those are some great points, even common bees. I was thinking on the West Coast Bombus occidentalis used to be a very common bee and now at least in Oregon, you really have to go up into the mountains to be able to find them, and the populations are very small. So that is a great example of [00:07:00] creating a baseline so that if we do see a decline, we can really measure it well.
[00:07:07] Well, so one thing that I'm really excited about, and I was really excited when I saw what you were working on is machine learning and natural history. It's not new, anybody who's used, iNaturalist and has used that "suggest species" option has learned that it has gotten pretty sophisticated. You go in, you take a picture of something and it kind of understands the pattern. And I guess it, recognizes it - I use this for plant identification all the time because I'm not a very good plant person. Can you tell me about some of the problems these machine learning systems encounter when it comes to bees?
[00:07:46] Brian Spiesmann: [00:07:46] So machine learning and bees - so bees are a difficult taxa to get a handle on for anybody even the experts struggle with many of the [00:08:00] uncommon species. Like in Andrena, and Dialictus there's a lot of just very difficult species that are hard to identify. Machine learning, I think is a good tool in that it can sort of learn its own features on its own. If you have a training data set, you have a data set of images that, you know for sure that they are all species "A" and you have another set that are all species "B". That's really all you need for the machine to figure out how to identify on its own what makes those different or what makes them similar so that you can differentiate between the two.
[00:08:43] So it comes up with its own feature set that may or may not be anything like what a human observer would use to identify the bee. So all it's using is pixels the digital numbers associated with the pixels [00:09:00] and some context within the image. And it uses that through this artificial neural network to generate these different scale features that it uses all of those. For some people it's a little bit of a black box. And so you don't know exactly what the features are that it's using to identify a species of bee.
[00:09:29] And a lot of times they're really abstract. So we really wouldn't be able to notice, it's not like it's saying, "oh, this one has three submarginal cells and this one has two." It doesn't know what a marginal cell is it doesn't know anything like that - it just knows that there's some pattern in the pixels that it could recognize. And so with iNaturalist it's using a set of models. There are a lot of models that have been developed by different organizations [00:10:00] like Google.
[00:10:02] There are these models that are developed to identify and differentiate sort of everyday items like dogs versus cats, spoons, cars, things like that. And it does pretty well on those types of problems. The models for bees and the ones that I'm using and the ones that iNaturalist I'm sure is using, are developed from those models. And they're sort of retrained to identify bees and plants and other organisms that iNaturalist does. And so when it's coming up with a sort of suggestion for what your image might be.
[00:10:43] The reason why iNaturalist doesn't do quite so good a job on species level bees is because it's trying, I think the way that their models do it, and I'm not exactly sure how their models work, but their models are designed [00:11:00] to look at whole range of taxas. So like, insects, mammals, fish, plants. And so it's using this very big model and so it doesn't have the resolution within the model to really get down and know at the species level what a particular bee might be.
[00:11:24] Whereas something like in the work that I've done, it's focused exclusively on well bumblebees in North America at this point. And so my model is very specific to this sort of taxonomic subset. And so it can get quite a bit better, I think than what iNaturalist is showing on their website. I'm sure they could get much better if they really focused on particular taxa at a time. It's just not totally clear how their models are actually working, but that's [00:12:00] sort of my guess.
[00:12:01] Andony Melathopoulos: [00:12:01] Okay. So I invited you here to talk about this specific initiative that you have the, Bee Machine platform and I guess the key thing here is that by really kind of focusing in on bees rather than the broad range of every creature from snails to carabid beetles then you're able to really kind of give that training a lot more examples. So tell us a little bit about what's involved with that. How do you train a machine to recognize bumblebees as you're doing?
[00:12:37] Brian Spiesmann: [00:12:37] Well, on my end it's pretty simple so I'm sort of building the hard work of a lot of other people, people that have developed the machine learning models, everyday people that are going out and capturing these images, the taxonomists that are doing important work of not [00:13:00] only identifying the bees, but telling us what makes a species a species. So all of this information I take and I take the images on iNaturalist, Bumblebee Watch, from BugGuide and I'm starting to get images from the Wisconsin Bumblebee Brigade.
[00:13:26] And so I take all of those images and they've all been identified many of them are identified by experts. And some of them, for example, from iNaturalist are agreed on by multiple people. And so some of which are the same experts that are identifying for some of these other groups. And so I'm taking these sort of pre-identified images and I feed them into my neural network model. And that's really, all I need to do, is I need to tell it, this image is from species "A" and I feed it through the [00:14:00] network and when the information gets passed through the network I know that it's supposed to be species "A", but the network may have told me that, "oh no, this was species "B".
[00:14:13] And so then it goes back, it passes back through the network, it rearranges and sort of remodels the links within that network, the weights of those links. And so it might change the network in such a way that when it runs through again it gets a little bit closer to the correct species and it makes that pass back and forth many, many times. And eventually the model arrives at the correct solution. And so if you do that for many, many images of all of the species that you're interested in and you pass those images back and forth through the network, and that's sort of the training process of making those connections between nodes within the network.
[00:14:56] Making them have the correct parameter [00:15:00] values to give you a correct answer. And then once you've trained the model, then you take brand new images that were never used to train the model and feed those through and see if you got the correct answer. And so that's the real test is to see if you can use brand new images to classify the image or identify the image of the bumblebee.
[00:15:21] Andony Melathopoulos: [00:15:21] Okay. And so you've been doing this and we'll put a link in the show notes. There's some great tips on how to take a picture of a bumblebee to be able to really optimize this machine learning platform to recognize the bee. But you've also done some of this validation work, which is up on the website. Tell us how it's fairing - this process of kind of going back. "No, no, no, that's not that" and then it goes back and learns. How is the machine doing?
[00:15:51] Brian Spiesmann: [00:15:51] It's doing pretty good. There's a lot of room for improvement and we're working on that sort of constantly. [00:16:00] So depending on who you are ask and you know what day it is, there's about 45 species of bumblebees in North America. So that's what Bee Machine is working on right now is working on identifying bumblebees. Eventually we want to get to a broader range of bees. So the dream way down the line is a sort of a general bee classifier. So we're focusing on North American bumblebees just as sort of a way to get a handle on the process. That's where most of the data is right now - we're taking the low hanging fruit.
[00:16:43] So of those 45 bumblebee species, we have enough data to train them all to classify 35 of those 45 species. And so those are basically the 35 most common bumblebees in North America. [00:17:00] So a general user would not likely come across those remaining 10 species. And those tend to be the Arctic species, species that occur where there just aren't a lot of people. And so that's one of the reasons why there aren't a lot of images of those is because there just aren't are a lot of people around to capture those images. And so of those 35 species our overall accuracy of the model is about 91%.
[00:17:31] And so that means if you go out into the field and you take a picture of the first bumblebee, you see the model will give you the correct identity about 91% of the time. And that's for the top 1%. So the way the model works is that it gives you the top predicted species, but it also gives you the second [00:18:00] most likely, the third most likely, you know, all way down to the bottom.
[00:18:03] And so it sort of ranks the likelihood of all of the species that you're going to see. And so in our web app Bee Machine, we give you the top three most likely species. And the top three accuracy is about 97-98%. So if you go out into the field and you capture an image of the first bumblebee you see our model will be about 97% accurate that it'll be one of those three species.
[00:18:36] Andony Melathopoulos: [00:18:36] Wow! Well, that's fantastic. And I guess some are hard to tell apart. I was really surprised that griseocollis and impatiens kind of look the same, but it easily can discern them. But here on the West coast, we've got a terrible complex between caliginosus and vosnesenskii where they're hard to tell a part, [00:19:00] and I noticed they kind of overlap. I imagine at some level, this gets you through, as you say, 90% of the differentiation allowing you to really kind of take the load off of the taxonomists who don't want to see another very, very common bumblebee.
[00:19:21] Brian Spiesmann: [00:19:21] And you're right, it does really well at some species. Like for example, Bombus affinis is the rusty patch bumblebee has one of the highest accuracies of all species.
[00:19:33] Andony Melathopoulos: [00:19:33] Oh how great!
[00:19:35] Brian Spiesmann: [00:19:35] Yeah. I think that's really great for conservation. So if you plug it into Bee Machine and it tells you that it's, Bombus affinis it's going to be Bombus affinis around 96% of the time. So you can be pretty sure that if you get that answer, that's what it is. And so for other things, like you mentioned some of the common [00:20:00] species, like Bombus griseocollis, Bombus impatiens, Bombus bimaculatus those are three species that new people to the field depending on the angle of the photo, those can be confused sometimes. And so things like that are really great because it takes the load off. It can take the load off taxonomists who can identify those with very little trouble. So having that sort of load off allows them to focus on the more difficult species, the species of greater concern. So I'm really happy about that aspect of it.
[00:20:47] Andony Melathopoulos: [00:20:47] I just learned about it just a couple months ago. When was it released and are you getting any feedback from people who are at that stage of just learning their bumblebees on [00:21:00] how they're finding it?
[00:21:02] Brian Spiesmann: [00:21:02] So I'm not getting a lot of feedback at this point. I'm seeing some use. And so I sort of monitor traffic on the website and I'm seeing use all over the United States and Canada where the bumblebees occur that my model is able to identify. But I'm seeing people from all over the world, just sort of checking in. I think there's a lot of curiosity just too, "what's the deal with this, is this really happening?" And I've had a little bit of feedback. So just for personal feedback, you mentioned some Facebook pages and people are posting bees to it.
[00:21:45] And so I've sort of, you know, snuck onto some of these Facebook pages and I helped identify some of the images up there. And there was a one woman who had [00:22:00] a picture of a Bombus affinis, a queen rusty patch. And she had planted this pollinator garden and specifically for attracting bees, I think she lived in Minnesota. So it was specifically for attracting rusty patch and so she was very happy to know that she was not only attracting the workers, but there was queens.
[00:22:24] And so I think people are starting to use it, I've got a little bit of feedback about people going back through old photos that they weren't able to identify on their own and plugging it in to Bee Machine. And it was sort of between one or two or three species that they couldn't really differentiate, but Bee Machine was really strongly favoring, one of those species. And so it gave them a little more confidence that it was species "A" instead of species "B".
[00:23:00] [00:23:00] Andony Melathopoulos: [00:23:00] That's fantastic. And that's a great story, I love the idea of the gardener in Minnesota, kind of getting that gratification and being able to know, "oh, I actually am achieving what I set out to do just by using this device." How do people get images in? How do people use it? It's on a website, right? So you're loading images in?
[00:23:22] Brian Spiesmann: [00:23:22] Right. So at this point it's a pretty simple interface. We're working on making the interface better all the time. So it's at beemachine.org and just type in beemachine.org, visit the website. There is a little bit of instructions on the website. So it tells you which species it's able to identify. It tells you a little bit about, you have to crop the image before you upload it. That's one of the things where it's a little bit of a hassle right now, but it's one of the things we're working on to be able to just to upload an image and not have to worry about cropping it.
[00:23:57] So if you crop your image right [00:24:00] close to the bee that reduces all of the extraneous pixels in the image, and it can really focus on the pixels associated with the bee and not all of that extra stuff in the background. Once you've uploaded it, you just press the upload button and the next page gives you the predictions and it tells you the top three predictions along with associated prediction probabilities.
[00:24:29] What I found through testing it myself over and over again, is that if it's really strongly favoring one species over the other two, like anywhere from like 95% or above, then I found that it's almost always that, that's the true identity. And the other ones are just there because those are the next closest species, even though they may be very [00:25:00] far from the actual identity. And so then you can click a link to just do a simple internet search on each of the species that comes up. So it could sort of, you know, jumpstart your research into, into saying, "okay, is this actually the species that it tells me it is?" So it's kind of simple at this point and we are actively working on making the user interface more little bit simpler and providing more educational information on what kind of bees you're actually seeing.
[00:25:42] Andony Melathopoulos: [00:25:42] There's some great information on there. And if there's anything that sort of marks a bee enthusiast, it's really wanting to see the next thing and really kind of be on board early. And so this is a great opportunity for people who are around the US because I can [00:26:00] imagine their information helps improve the program and its usability. And so they're actually contributing in some small way to making it even better each month.
[00:26:15] Brian Spiesmann: [00:26:15] Yeah, definitely. And that's going to be much more true in the future. So one of the things we're planning on is actually using the images that people upload to sort of continuously retrain the models. So the more images people upload, the better the model will be in terms of the classifications. So the feedback I receive is great, but then eventually it's going to be directly contributing to the quality of the model. And I'd also like to plug in this sort of machine learning capability into broader monitoring [00:27:00] projects. So I think it'll be really helpful for getting the broader community of bee enthusiast researchers out there able to quickly and accurately get a good picture of what's going on with bees.
[00:27:23] Andony Melathopoulos: [00:27:23] Well, let's take a quick break and I want to actually pick up on that point, just how machine learning can be sort of part of the larger division of labor, in terms of really surveying our bees across the state. So let's take a quick break and we'll be right back.
[00:27:52] Okay. So as we're back. And so I really was curious, you mentioned earlier that the machine, is just looking at pixels and it's just [00:28:00] picking things up. It has not gone through the classical keys, the genus or species keys. So as a taxonomist, are you finding that what the machine is picking up on are features that never occurred to you as being diagnostic?
[00:28:17] Brian Spiesmann: [00:28:17] Yeah, so it's interesting. So, small clarification, I wouldn't really consider myself a taxonomist. I consider myself an ecologist that really relies on taxonomists and I'm sort of an amateur taxonomist that can get myself into trouble if I'm not careful.
[00:28:38] Andony Melathopoulos: [00:28:38] Okay.
[00:28:39] Brian Spiesmann: [00:28:39] So with the machine, it's definitely making similar mistakes that the human observer would make. The machine is thinking that Bombus [00:29:00] impatiens is more similar looking to Bombus bimaculatus than it is to Bombus pensylvanicus. So it's seeing bees that we think would be more similar looking species that we think would be similar are similar and species that we think would be different are different. The particular characters that it's using are kind of a mystery to me. I haven't dug too deep into the layers in the neural network, which can tell you which kind of features it's looking at any in these different layers.
[00:29:42] And so as you move through a network, the early layers are very fine features, like say for example, you were trying to identify faces. The first layers would be looking at fine scale features like eyes and [00:30:00] noses and ears. And then the middle areas, would be looking at more developed things like combinations of eyes and like parts of your face. And then the very end would be looking at the whole face in general.
[00:30:15] And what that means for bees, I haven't sort of dug into the model to see how that's coming out. But I did do that a little bit for a previous model when I was looking at just bee wings and that was interesting because you can take the image that you plug in and then sort of overlay heat map on it. And the heat map tells you the areas that were more important than other areas for the machine to classify the image. So like a bright red area, would be very important for the machine. And then the blue area would be not so important, it doesn't really use that area.
[00:30:52] So the interesting thing about that was that it was on just this image of a wing, it was focusing on an area of the [00:31:00] wing that taxonomists probably wouldn't use to identify what that was. It wasn't looking at the curvature of the marginal cells, and then the number of submarginal cells. It was looking at the leading edge of the wing and for this genus, which I think it was Agapostemon in this example, it was just looking at something that a taxonomist just wouldn't use. And so in that case that's sort of an example of how the machine generates its own feature set.
[00:31:31] It doesn't necessarily use what we use, which could be important down the road, or it could be at least useful down the road to help us understand when two species are actually different based on these morphological features. So that's definitely something that needs to be worked out, but there's a possibility that it could be useful for differentiating species or [00:32:00] maybe finding characters that weren't noticeable before or maybe we just didn't notice them. Or it could just be something in the pixels, something that we'd never be able to get all.
[00:32:12] Andony Melathopoulos: [00:32:12] You know, it reminds me, remember back after the cold war they had that guy play the IBM computer in chess. The computer was like, "oh yeah, I can hold my own." And it sort of raises the question, there has been the shortage of native bee taxonomy across the US we really have not invested a lot in making sure those positions are funded and people are there. And I can imagine this kind of technology could compliment their work. How do you envision that kind of division of labor between professional taxonomists, who are constantly working at the very fine gradations with the machine learning?
[00:32:58] Brian Spiesmann: [00:32:58] Yeah, I think they could [00:33:00] work nicely together. So this technology won't replace taxonomists. We need taxonomists to help us understand differences between species to help revise our understanding of what makes this population different from that population? Are they different species? What makes them different? That is something that at least now this machine learning technology can't do, what it can do is take that information from taxonomists and once a taxonomists or an expert has identified a species of bee, then we can use that image to train a model that non-experts can use to identify their own species.
[00:33:54] So in that case I think it could be really useful and really [00:34:00] important for sort of taking the load off of taxonomists. In terms of making it so they can reduce their time identifying the common species and focus more on the more challenging species. I think it could be very complimentary to what they're doing. So if the taxonomists is really focused on some aspects of taxonomic research, working on a revision, if they are spending more of their time identifying common species that get sent to them, then it takes away from some of their research.
[00:34:50] But then again you mentioned that there's a lack of funding for taxonomists. And so they get a lot of their funding from identifying [00:35:00] these bee specimens that get sent to them. So this is not meant to replace them, I think this technology is meant to help lessen the burden on them. There's also just more specimens, more images out there than they can handle right now. Especially on talking with people at Bumblebee Watch they have a big backlog of images that have yet to be identified.
[00:35:35] And those taxonomists, those experts there are donating a lot of their time to do this and it can be a challenge for them to get through a lot of these - literally thousands of images to be identified. And so if that could lessen their load and in that regard, it would make more data available for researchers to understand the trends in bee [00:36:00] population dynamics. And in bee range shifts and things like that. And so more data on some of these big sort of community science driven data sets like Bumblebee Watch, iNaturalist, and BugGuide.
[00:36:16] So it would provide more data to understand bees, it would provide a sort of relief to taxonomists to focus on the things that they need to get done and could also be really useful as an educational tool for people and get people more interested in contributing some of these images. If they get feedback right away on the identity of the bee that they upload then it could help, bring a little more enthusiasm to the field and make people feel like they're contributing a little bit more.
[00:36:58] Andony Melathopoulos: [00:36:58] Fantastic. Well, let's [00:37:00] take a quick break. We'll come back. Our last segment where we ask you for some recommendations for our listeners. Okay. So we'll be back in just a second!
[00:37:26] Okay. We're back. I know you're on the hot seat now. We got three questions for you. The first one is a book recommendation.
[00:37:34] Brian Spiesmann: [00:37:34] So the book that I've been using a lot of to help develop my models, develop my understanding of bumblebees in North America is Bumblebees of North America. It's a great sort of field guide, a great tool to take out. And I've really used it to help with my understanding of bumblebee taxonomy, and where [00:38:00] these species live, what makes them different from other bumblebees.
[00:38:03] Andony Melathopoulos: [00:38:03] It's a fantastic book it's really affordable. Anybody can pick it up and it really does kind of show you how to identify. I mean I guess this would be one of the tricky things is it's one thing to take queens and be able to recognize them, but the males get to be a little bit hard to identify and the guide has some great photos. It allows you to sort of see the color patterns, but it also has that fantastic genitalia descriptors. So if you're really hardcore a good way to identify males is with those genitalium images.
[00:38:43] Okay. Well, that's a great recommendation. Thanks for recommending it. And I really encourage people to pick it up if you're interested in bumblebees, it's a great compliment as you're kind of using Bee Machine to learn to have your copy beside you. Alright. Our next question, is there a go to tool that you really [00:39:00] rely on?
[00:39:01] Brian Spiesmann: [00:39:01] Yeah. So when I'm out in the field sampling I usually have two tools. One tool that is the goto tool for everybody is a collecting net. But the other thing that I use quite a lot is that is what I call a beevac. I don't know if that's what everybody else calls it, basically it's a modified dustbuster where you can use it to suck up bees and it nicely brings them into little capsules that you can keep bees separate or load up a new capsule and start catching more bees. So it's a great tool that I use whenever I'm out in the field catching bees!
[00:39:44] Andony Melathopoulos: [00:39:44] I was out with Rich Hatfield once with all the Bumblebee Watches across the US and for them they actually cool their bees down before they take a picture. And imagine if you have something like a beevac and a [00:40:00] little ice chest, you can get some really crisp images for submitting.
[00:40:04] Brian Spiesmann: [00:40:04] Yeah. It's great. You just, you suck it up, you pop out the little capsule and you can put it in the ice chest and cool down for a few minutes and yeah, it's great, I use it for sampling. And it's great if you want to keep observations separate, like you're focusing on one host plant. And you just get all the bees into that capsule from that host plant over some period of time, and then you switch to another one, you pop in a new capsule, and that way you can keep all of your samples together nicely.
[00:40:35] Andony Melathopoulos: [00:40:35] Fantastic. Well, our last question for you is, do you have a favorite pollinator species?
[00:40:41] Brian Spiesmann: [00:40:41] I like a lot of pollinators! I think it's not a very original one, it's kind of boring, but I really like Bombus impatiens the common eastern bumblebee. It's everywhere, it's easy to identify, it's just sort of uncomfortable known [00:41:00] bee.
[00:41:01] Andony Melathopoulos: [00:41:01] It's a great one. I always love having bees that a beginner can identify right away. Because it really does help your confidence, you're all nervous, and you're like, "I can tell a species, I got it." That was probably was one of the first species when I was in Prince Edward Island doing my graduate work that I really kind of cottoned onto as the first one that I noticed as distinct.
[00:41:27] Well, thank you so much for taking time. We're really looking forward to the development of this technology. I think it's going to really help a lot for a lot of people who are just starting out and trying to learn how to identify bumblebees. And it sounds like it's going to have some great impact in terms of being able to survey bees.
[00:41:45] Brian Spiesmann: [00:41:45] Yeah, I hope so. We're developing all the time or we're have a mobile app in the works that people are going to be able to take out on their mobile phone and take images and quickly get answers [00:42:00] back. And, we hope to link it with databases so that you can use it for field work, link it to your databases associated with your own project. Link the modeling structure in with that sort of field deployable hardware that you can put out as sort of automated monitoring over long periods of time. So we have a lot in the works here and we're pretty excited to see where it leads.
[00:42:30] Andony Melathopoulos: [00:42:30] Well, thank you so much! I'm so excited. I'm looking forward to catching up with you next year, maybe. Take care.
[00:42:41] Thank you so much for listening. The show is produced by Quinn Sinanan Neal who's a student here at OSU in the New Media Communications Program and the show wouldn't even be possible without the support of the Oregon Legislature, the Foundation for Food and Agricultural Research and Western Sara. Show notes with links mentioned on each episode are available on [00:43:00] the website, which is at pollinationpodcast.oregonstate.edu.
[00:43:05] I also love hearing from you and there's several ways to connect with me. The first one is you can visit the website and leave an episode specific comment, you can suggest a future guest or topic, or ask a question that could be featured in a future episode. But you can do the same things on Twitter, Instagram, or Facebook, but visiting the Oregon Bee Project. Thanks so much for listening and see you next week!
Identifying bees is notoriously difficult and often is an obstacle to beginners interested in contributing to native bee surveys. In this episode we learn about a new initiative to identify bees with machine learning.
Dr. Spiesman is a professor in the Department of Entomology at Kansas State University. His lab's research focuses on pollinator ecology and conservation while developing tools using AI and computer vision for automated bee identification and observation.
Links Mentioned:
Book recommendation:
Richardson, L. L., Williams, P. H., Thorp, R. W., Colla, S. R. (2014). Bumble Bees of North America: An Identification Guide. United Kingdom: Princeton University Press.
Go-To-Tools:
- Collecting net
- Bee vacuum
Favorite Pollinator: Bombus impatiens
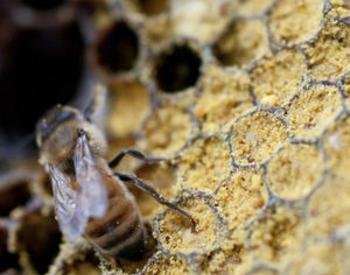